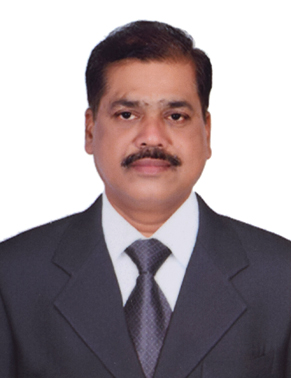
Contact
Mobile : +91-9952654338
e-mail : kseethadde@yahoo.com kseethaddeau@gmail.com
Address
Department of Computer & Information Science,
Annamalai University,
Annamalai Nagar,
Chidambaram – 608 002,
Tamil Nadu, INDIA.
|
JOURNALS
1.
|
Selvakumar, V., and K. Seetharaman. (2022). HOML-SL: IoT Based Early Disease Detection and Prediction for Sugarcane Leaf using Hybrid Optimal Machine Learning Technique, Journal of Survey in Fisheries Sciences 10(2S), 3284-3309 (Scopus Indexed). [ ]
|
2.
|
Selvakumar, V, and K. Seetharaman. (2022). HML-SL: A hybrid machine learning technique for sugarcane leaf disease detection, NeuroQuantology, 20(19), 1605-1629, doi: 10.48047/nq.2022.20.19.NQ99145, (SCI Indexed), (SCI Indexed). [ ]
|
3.
|
ABSTRACT:
The development of digital technology is utilized by people to capture and share video frames. At present, rather than capturing images, people are interested in recording video footage for exploring information. Here, retrieval of video from large databases is challenging due to the continuous frame count. To overcome these challenges associated with the retrieval of video from available databases, this research proposed a likelihood-based regression approach for video processing. To improve the retrieval accuracy of video sequences, the proposed method utilizes a likelihood estimation technique integrated with a regression model. The likelihood estimate measures the pixel level roughly for estimating the pixel range, after which the regression approach measures the pixel level for transforming certainly blurred and unwanted pixels. In the proposed likelihood regression approach, the video is converted into a video frame and stored in a database. Query frames are taken into account by the generated database depending on the features which are used for a given video to be retrieved. The significant video retrieval performance obtained from the simulation results for the proposed likelihood-based regression model shows that the proposed model performs well over the other state-of-the-art techniques.
Sathiyaprasad, B., and K. Seetharaman. (2022). Unsupervised learning-based recognition and extraction for intelligent automatic video retrieval, The Photogrammetric Record, doi.org/10.1111/phor.12427 (ISSN: 1477-9730 – SCI Indexed). [ ]
|
4.
|
ABSTRACT:
The development of digital technology is utilized by people to capture and share video frames. At present, rather than capturing images, people are interested in recording video footage for exploring information. Here, retrieval of video from large databases is challenging due to the continuous frame count. To overcome these challenges associated with the retrieval of video from available databases, this research proposed a likelihood-based regression approach for video processing. To improve the retrieval accuracy of video sequences, the proposed method utilizes a likelihood estimation technique integrated with a regression model. The likelihood estimate measures the pixel level roughly for estimating the pixel range, after which the regression approach measures the pixel level for transforming certainly blurred and unwanted pixels. In the proposed likelihood regression approach, the video is converted into a video frame and stored in a database. Query frames are taken into account by the generated database depending on the features which are used for a given video to be retrieved. The significant video retrieval performance obtained from the simulation results for the proposed likelihood-based regression model shows that the proposed model performs well over the other state-of-the-art techniques.
Mahendran, T., and K. Seetharaman. (2022). Video sequence feature extraction and segmentation using likelihood regression model, Journal of Positive School Psychology, 6(10), 2553-2562. (SCI Indexed). [ ]
|
5.
|
ABSTRACT:
The development of digital technology is utilized by people to capture and share video frames. At present, rather than capturing images, people are interested in recording video footage for exploring information. Here, retrieval of video from large databases is challenging due to the continuous frame count. To overcome these challenges associated with the retrieval of video from available databases, this research proposed a likelihood-based regression approach for video processing. To improve the retrieval accuracy of video sequences, the proposed method utilizes a likelihood estimation technique integrated with a regression model. The likelihood estimate measures the pixel level roughly for estimating the pixel range, after which the regression approach measures the pixel level for transforming certainly blurred and unwanted pixels. In the proposed likelihood regression approach, the video is converted into a video frame and stored in a database. Query frames are taken into account by the generated database depending on the features which are used for a given video to be retrieved. The significant video retrieval performance obtained from the simulation results for the proposed likelihood-based regression model shows that the proposed model performs well over the other state-of-the-art techniques.
Subramanyam Rallabandi, V.P., and K. Seetharaman. (2022). Deep learning-based classification of healthy aging controls, mild cognitive impairment and Alzheimer’s disease using fusion of MRI-PET imaging, Biomedical Signal Processing and Control, 80, 104312, https://doi.org/10.1016/j.bspc.2022. (SCI Indexed). [ ]
|
6.
|
ABSTRACT:
The development of digital technology is utilized by people to capture and share video frames. At present, rather than capturing images, people are interested in recording video footage for exploring information. Here, retrieval of video from large databases is challenging due to the continuous frame count. To overcome these challenges associated with the retrieval of video from available databases, this research proposed a likelihood-based regression approach for video processing. To improve the retrieval accuracy of video sequences, the proposed method utilizes a likelihood estimation technique integrated with a regression model. The likelihood estimate measures the pixel level roughly for estimating the pixel range, after which the regression approach measures the pixel level for transforming certainly blurred and unwanted pixels. In the proposed likelihood regression approach, the video is converted into a video frame and stored in a database. Query frames are taken into account by the generated database depending on the features which are used for a given video to be retrieved. The significant video retrieval performance obtained from the simulation results for the proposed likelihood-based regression model shows that the proposed model performs well over the other state-of-the-art techniques.
Subramanyam Rallabandi, V.P., and K. Seetharaman. (2022). Classification of cognitively normal controls, mild cognitive impairment and Alzheimer’s disease using transfer learning approach, Biomedical Signal Processing and Control, 79, https://doi.org/10.1016/j.bspc.2022.104092. (SCI Indexed). [ ]
|
7.
|
ABSTRACT:
The development of digital technology is utilized by people to capture and share video frames. At present, rather than capturing images, people are interested in recording video footage for exploring information. Here, retrieval of video from large databases is challenging due to the continuous frame count. To overcome these challenges associated with the retrieval of video from available databases, this research proposed a likelihood-based regression approach for video processing. To improve the retrieval accuracy of video sequences, the proposed method utilizes a likelihood estimation technique integrated with a regression model. The likelihood estimate measures the pixel level roughly for estimating the pixel range, after which the regression approach measures the pixel level for transforming certainly blurred and unwanted pixels. In the proposed likelihood regression approach, the video is converted into a video frame and stored in a database. Query frames are taken into account by the generated database depending on the features which are used for a given video to be retrieved. The significant video retrieval performance obtained from the simulation results for the proposed likelihood-based regression model shows that the proposed model performs well over the other state-of-the-art techniques.
Kumar, B.S., and K. Seetharaman. (2022). Content based video retrieval using deep learning feature extraction by modified VGG_16, Journal of Ambient Intelligence and Humanized Computing, 1-13, https://doi.org/10.1007/s12652-022-03869-y. (SCI Indexed). [ ]
|
8.
|
ABSTRACT:
The development of digital technology is utilized by people to capture and share video frames. At present, rather than capturing images, people are interested in recording video footage for exploring information. Here, retrieval of video from large databases is challenging due to the continuous frame count. To overcome these challenges associated with the retrieval of video from available databases, this research proposed a likelihood-based regression approach for video processing. To improve the retrieval accuracy of video sequences, the proposed method utilizes a likelihood estimation technique integrated with a regression model. The likelihood estimate measures the pixel level roughly for estimating the pixel range, after which the regression approach measures the pixel level for transforming certainly blurred and unwanted pixels. In the proposed likelihood regression approach, the video is converted into a video frame and stored in a database. Query frames are taken into account by the generated database depending on the features which are used for a given video to be retrieved. The significant video retrieval performance obtained from the simulation results for the proposed likelihood-based regression model shows that the proposed model performs well over the other state-of-the-art techniques.
Suresh, G., and K. Seetharaman. (2022). Real-time automatic detection and classification of groundnut leaf disease using hybrid machine learning techniques, Multimedia Tools and Applications, 1-29. https://doi.org/ 10.1007/s11042-022-12893-1. (SCI Indexed). [ ]
|
9.
|
ABSTRACT:
The development of digital technology is utilized by people to capture and share video frames. At present, rather than capturing images, people are interested in recording video footage for exploring information. Here, retrieval of video from large databases is challenging due to the continuous frame count. To overcome these challenges associated with the retrieval of video from available databases, this research proposed a likelihood-based regression approach for video processing. To improve the retrieval accuracy of video sequences, the proposed method utilizes a likelihood estimation technique integrated with a regression model. The likelihood estimate measures the pixel level roughly for estimating the pixel range, after which the regression approach measures the pixel level for transforming certainly blurred and unwanted pixels. In the proposed likelihood regression approach, the video is converted into a video frame and stored in a database. Query frames are taken into account by the generated database depending on the features which are used for a given video to be retrieved. The significant video retrieval performance obtained from the simulation results for the proposed likelihood-based regression model shows that the proposed model performs well over the other state-of-the-art techniques.
Seetharaman, K., and Mahendran, T. (2022). Leaf Disease Detection in Banana Plant using Gabor Extraction and Region-Based Convolution Neural Network (RCNN), Journal of Institution of Engineers (India) Series A, https://doi.org/10.1007/s40030-022-00628-2. (SCI Indexed) [ ]
|
10.
|
ABSTRACT:
The development of digital technology is utilized by people to capture and share video frames. At present, rather than capturing images, people are interested in recording video footage for exploring information. Here, retrieval of video from large databases is challenging due to the continuous frame count. To overcome these challenges associated with the retrieval of video from available databases, this research proposed a likelihood-based regression approach for video processing. To improve the retrieval accuracy of video sequences, the proposed method utilizes a likelihood estimation technique integrated with a regression model. The likelihood estimate measures the pixel level roughly for estimating the pixel range, after which the regression approach measures the pixel level for transforming certainly blurred and unwanted pixels. In the proposed likelihood regression approach, the video is converted into a video frame and stored in a database. Query frames are taken into account by the generated database depending on the features which are used for a given video to be retrieved. The significant video retrieval performance obtained from the simulation results for the proposed likelihood-based regression model shows that the proposed model performs well over the other state-of-the-art techniques.
Kalaivani, S., and K. Seetharaman. (2022). A three-stage ensemble boosted convolutional neural network for classification and analysis of COVID-19 chest X-ray images, International Journal of Cognitive Computing in Engineering, 3, 35-45. (Scopus Indexed). [ ]
|
11.
|
ABSTRACT:
The development of digital technology is utilized by people to capture and share video frames. At present, rather than capturing images, people are interested in recording video footage for exploring information. Here, retrieval of video from large databases is challenging due to the continuous frame count. To overcome these challenges associated with the retrieval of video from available databases, this research proposed a likelihood-based regression approach for video processing. To improve the retrieval accuracy of video sequences, the proposed method utilizes a likelihood estimation technique integrated with a regression model. The likelihood estimate measures the pixel level roughly for estimating the pixel range, after which the regression approach measures the pixel level for transforming certainly blurred and unwanted pixels. In the proposed likelihood regression approach, the video is converted into a video frame and stored in a database. Query frames are taken into account by the generated database depending on the features which are used for a given video to be retrieved. The significant video retrieval performance obtained from the simulation results for the proposed likelihood-based regression model shows that the proposed model performs well over the other state-of-the-art techniques.
Mahendran, T., and K. Seetharaman. (2021). Detection of Disease in Banana Fruit using Gabor Based Binary Patterns with Convolution Recurrent Neural Network, Turkish Online Journal of Qualitative Inquiry (TOJQI), 12 (9), 6958-6966. (SCI Indexed) [ ]
|
12.
|
ABSTRACT:
The development of digital technology is utilized by people to capture and share video frames. At present, rather than capturing images, people are interested in recording video footage for exploring information. Here, retrieval of video from large databases is challenging due to the continuous frame count. To overcome these challenges associated with the retrieval of video from available databases, this research proposed a likelihood-based regression approach for video processing. To improve the retrieval accuracy of video sequences, the proposed method utilizes a likelihood estimation technique integrated with a regression model. The likelihood estimate measures the pixel level roughly for estimating the pixel range, after which the regression approach measures the pixel level for transforming certainly blurred and unwanted pixels. In the proposed likelihood regression approach, the video is converted into a video frame and stored in a database. Query frames are taken into account by the generated database depending on the features which are used for a given video to be retrieved. The significant video retrieval performance obtained from the simulation results for the proposed likelihood-based regression model shows that the proposed model performs well over the other state-of-the-art techniques.
Sathiyaprasad, B., , and K. Seetharaman. (2021). Medical Surgical Video Recognition and Retrieval Based on Novel Unified Approximation, Journal of Medical Imaging and Health Informatics, 11(11), pp. 2733-2746; DOI: https://doi.org/10.1166/jmihi.2021.3874; (ISBN: 2156-7018; SCI Indexed). [ ]
|
13.
|
ABSTRACT:
The development of digital technology is utilized by people to capture and share video frames. At present, rather than capturing images, people are interested in recording video footage for exploring information. Here, retrieval of video from large databases is challenging due to the continuous frame count. To overcome these challenges associated with the retrieval of video from available databases, this research proposed a likelihood-based regression approach for video processing. To improve the retrieval accuracy of video sequences, the proposed method utilizes a likelihood estimation technique integrated with a regression model. The likelihood estimate measures the pixel level roughly for estimating the pixel range, after which the regression approach measures the pixel level for transforming certainly blurred and unwanted pixels. In the proposed likelihood regression approach, the video is converted into a video frame and stored in a database. Query frames are taken into account by the generated database depending on the features which are used for a given video to be retrieved. The significant video retrieval performance obtained from the simulation results for the proposed likelihood-based regression model shows that the proposed model performs well over the other state-of-the-art techniques.
Pooranachandranm C., Chembian, W.T., and K. Seetharaman. (2021). ). Image Retrieval Based on Adaptive Gaussian Markov Random Field Model with Bayes Back-propagation Neural Network, SN Computer Science, 3(31), pp. 1-17; (Springer Nature; ISSN: 2662-995X; Scopus Indexed). [ ]
|
14.
|
ABSTRACT:
The development of digital technology is utilized by people to capture and share video frames. At present, rather than capturing images, people are interested in recording video footage for exploring information. Here, retrieval of video from large databases is challenging due to the continuous frame count. To overcome these challenges associated with the retrieval of video from available databases, this research proposed a likelihood-based regression approach for video processing. To improve the retrieval accuracy of video sequences, the proposed method utilizes a likelihood estimation technique integrated with a regression model. The likelihood estimate measures the pixel level roughly for estimating the pixel range, after which the regression approach measures the pixel level for transforming certainly blurred and unwanted pixels. In the proposed likelihood regression approach, the video is converted into a video frame and stored in a database. Query frames are taken into account by the generated database depending on the features which are used for a given video to be retrieved. The significant video retrieval performance obtained from the simulation results for the proposed likelihood-based regression model shows that the proposed model performs well over the other state-of-the-art techniques.
Amutha, A., and K. Seetharaman. Ashish, A., (2021). Cyber Security by Prediction of Time Synchronization using Bayesian Base Gradient Descent Approach, Journal of Scientific & Industrial Research, 80, 347-353 (SCI Indexed; IF: 1.056). (SCI Indexed) [ ]
|
15.
|
ABSTRACT:
The recent challenge faced by the users from the multimedia area is to collect the relevant object or unique image from the collection of huge data. During the classification of semantics, the media was allowed to access the text by merging the media with the text or content before the emergence of content based retrieval. The identification of this feature has become major challenges, so to overcome this issue this paper focuses on a deep learning technique with maximum likelihood regression (MLR) model for segmentation and Feature extraction of the input video. Likelihood estimation is to roughly measure the level of pixel, and then regression method determines pixel level to certainly transformblurred and unwanted pixels. The segmentation is done based on the likelihood estimation and the feature of this segmented video is extracted using Modified_ VGG-16 (M_VGG-16) architecture. The result of this technique has been compared with the existing other feature extraction techniques such as conventional Histogram of Oriented Gradients (HOG), LBP (Local Binary Patterns) and CNN (Convolution Neural Network) methods. In this scheme the video frame image retrieval is performed by assigning the indexing to the all video files in the database in order to perform the system more efficiently. Thus the system produces the top result matches for the similar query in comparison with the existing techniques based on precision of 90%, recall of 93% and F1 score of 91% in optimized video frame retrieval.
Satheesh Kumar, B., and K. Seetharaman. (2021). Segmentation And Feature Extraction Of Content Based Video Retrieval Using Maximum Likelihood Regression Model With Modified-Vgg-16, Webology, 18(6):1735-188. ISSN: 1735-188X (Scopus Indexed) [ ]
|
16.
|
ABSTRACT:
The development of digital technology is utilized by people to capture and share video frames. At present, rather than capturing images, people are interested in recording video footage for exploring information. Here, retrieval of video from large databases is challenging due to the continuous frame count. To overcome these challenges associated with the retrieval of video from available databases, this research proposed a likelihood-based regression approach for video processing. To improve the retrieval accuracy of video sequences, the proposed method utilizes a likelihood estimation technique integrated with a regression model. The likelihood estimate measures the pixel level roughly for estimating the pixel range, after which the regression approach measures the pixel level for transforming certainly blurred and unwanted pixels. In the proposed likelihood regression approach, the video is converted into a video frame and stored in a database. Query frames are taken into account by the generated database depending on the features which are used for a given video to be retrieved. The significant video retrieval performance obtained from the simulation results for the proposed likelihood-based regression model shows that the proposed model performs well over the other state-of-the-art techniques.
Kumar, B.S., and K. Seetharaman. (2021). Video sequence feature extraction and segmentation using likelihood regression model, Multimedia Tools and Applications, https://doi.org/10.1007/s11042-021-10829-9(SCI Indexed). [ ]
|
17.
|
ABSTRACT:
Coordinated Universal Time (UTC) is based on the biggest possible number of atomic clocks of various categories, to be found in various regions of the world and connected through a network which allows precise time comparisons amid remote sites. In India, UTC system is followed and cyber security issues are a concern. This research explains the security problems faced with UTC (k) system and describes how enhancement rectifies such problem. There is necessity for single time scale for whole nation. This research adopted qualitative approach and experimental design for carrying out investigation. Data are collected from National Physical Laboratory and National measurement institute of India. Proposed Software to be used in this particular research for implementing the framework is archimate open source. The aim of intention of this research is to design and develop cyber physical security framework for national time dissemination. Security problems are rectified with developed cyber physical security framework. The developed cyber security framework achieves traceability and synchronization in cyber security environment. This research would be helpful for practitioners, academicians, policy-makers, capitalists to understand the need for developing a framework for national time dissemination in cyber-secure environment.
Amutha. A., and K. Seetharaman. and Agarwal, A. (2021). Design and Development of a Cyber Security Framework for National Time Dissemination, SN Computer Science 2, 77. https://doi.org/10.1007/s42979-021-00471-5 (Scopus Indexed). [ ]
|
18.
|
ABSTRACT:
This paper proposes a novel method, which is coined as ARBBPNN, for biometric-oriented face detection, based on autoregressive model with Bayes backpropagation neural network (BBPNN). Firstly, the given input colour key face image is modelled to HSV and YCbCr models. A hybrid model, called HS-YCbCr, is formulated based on the HSV and YCbCr models. The submodel, H, is divided into various sliding windows of size, 3 9 3. The model parameters are estimated for the window using the BBPNN. Based on the model coefficients, autocorrelation coefficients (ACCs) are computed. An autocorrelation test tests the significance of the ACCs. If the ACC passes the test, then it is inferred that the small image region, viz. the window, represents the texture and it is treated as the texture feature. Otherwise, it is regarded as structure, which is treated as the shape feature. The texture and shape features are formulated as feature vectors (FV) separately, and they are combined into a single FV. This process is performed for all colour submodels. The FVs of the submodels are combined into a single holistic vector, which is treated as the FV of the key face image. The key FV has twenty feature elements. The similarity of the key and target face images is examined, based on the key and target FVs, by deploying multivariate parametric statistical tests. If the FVs of the key and target images pass the tests, then it is concluded that the key and target face images are the same; otherwise, they are regarded as different. The GT, FSW, Pointing'04, and BioID datasets are considered for the experiments. In addition to the above datasets, we have constructed a dataset with face images collected from Google, and many images captured through a digital camera. It is also subjected to the experiment. The obtained recognition results show that the proposed ARBBPNN method outperforms the existing methods.
Vasanthi, M. and K. Seetharaman. (2021). A Hybrid Method for Biometric Authentication Oriented Face Detection Using Autoregressive Model with Bayes Backpropagation Neural Network, Soft Computing, 25, 1659-1680 https://doi.org/10.1007/s00500-020-05500-8 (ISSN: 1432-7643;SCI Indexed). [ ]
|
19.
|
ABSTRACT:
This paper proposes a method for biometric-driven facial image recognition, based on multivariate correlation analysis, which extracts geometrical feature points and low-level visual features. The low-level visual features, such as colour and texture, are extracted at local from the selected prominent regions of the facial image. The geometrical features are captured using the Active Shape Model (ASM). The colour features are extracted from the YCbCr colour model, and the autocorrelation method is deployed to extract the texture features. The extracted features are formed as a feature tensor matrix. The feature matrix of the key face image is compared to the feature matrices of the target face image that are stored in the feature vector database using the Canonical Correlation method. The correlation between the key and target feature matrices is tested, whether it is highly significant or not. If it is significantly correlated, then it is inferred that the key and target face images are the same; otherwise, it is concluded that they are different. The benchmark facial image datasets, GT, LFW, and Pointing '04 datasets, considered for the experiments; in addition to the datasets, a facial image database has been constructed with celebrities on our interest which has also been subjected to experiments. The proposed method resulted in a mean precision score (mP@a) of 95.27%, 94.20%, 96.19%, and 96.05 for GT, LFW, Pointing '04, and our datasets, respectively. Also, the F-score was calculated that are, 96.78%, 95.15%, 97.08%, and 96.96% for GT, LFW, Pointing '04, and our datasets, respectively. The results obtained by the proposed method are comparable to the existing methods.
Vasanthi, M. and K. Seetharaman. (2021). Facial Image Recognition for Biometric Authentication Systems Using A Combination of Geometrical Feature Points and Low-level Visual Features, Journal of King Saud University - Computer and Information Sciences, https://doi.org/10.1016/j.jksuci.2020.11.028, (ISSN: 1319-1578; SCI Indexed). [ ]
|
20.
|
ABSTRACT:
This paper presents an Advanced Stochastic Gradient Descent (A-SGD) with Moving-Equivalence Class Clustering and indefinite quantity Lattice Traversal (MECQLT) algorithm. The Moving-Equivalence Class Clustering and indefinite quantity Lattice Traversal (MECQLT) algorithm identified as a reiterative (repetitive movement) procedure, which is used to find the video gesture of a continuous streaming video, that insignificant to the retrieval time to a feasible extent. However, the above Video Manifestation Identification (VMI) takes the input from the feature of vide file; this might be extracted from the video input frame. Hierarchical Softmax Stored Counterstrike (HSC) Algorithm is used to make the Feature extraction in video retrieval by using Meet at a point-framing. These ideas implemented for a recovery purpose by attaining the relationship among the nearby region of the video frame itself. For these categories, the two shortest distance of vertical or horizontal nearby region frame is examined. After computing the A-SGD, the noise-less feature frame is found by the MECQLT algorithm. Finally, the proposed method shows the relevance of algorithms for automatic recognition of fine-tuned video file in the streaming video, on the automatic recognition carried out by parsing, localization, normalization and segmentation procedures. The implementation of the fine-tuned video of a streaming video and their relations in making up the error rate is unused and neglected. This neglected error rate shows that the nature of the change in the values of the parameters depends on the streaming video frame length. It is proposed to carry out an individual assessment of the reliability of the Framing mechanism for a block of time frame information.
Sathiyaprasad, B. and K. Seetharaman. (2019). Text-Image Queries based Video Retrieval using Image Ontology Queries Formation, Journal of Advanced Research in Dynamical & Control Systems, Vol. 12(12), pp. 1750-1769 (ISSN: 1943-023X; Scopus Indexed, h-index 8). [ ]
|
21.
|
ABSTRACT:
This article proposes a novel method, based on the multivariate parametric statistical tests of hypotheses, which classifies thenormal skin lesion images and the various stages of the melanoma images. The melanoma images are categorized into two classes,such as initial stage and advanced stage, based on the degree of aggressiveness of the cancer. The region of interest is identified andsegmented from the input skin melanoma image. The features, such as HSV color, shape, and texture, are extracted from the regionof interest. The features are treated as a feature space, which is assumed to be a multivariate normal random field. The proposedstatistical tests are employed to identify and classify the melanoma images. The proposed method yields an average correctclassification up to 92.67% for the normal skin lesion versus the initial and the advanced stage of the melanoma images; up to91.67% for initial stage melanoma versus the normal skin lesion and the advanced stage melanoma; and up to 92.57% for theadvanced stage melanoma versus the normal skin lesion and the initial stage melanoma. The proposed method yields better results.
K. Seetharaman, (2019).Melanoma Image Classification Based on Color, Shape, and Texture Features Using Multivariate Statistical Tests: Journal of Computational and Theoretical Nanoscience, 16(4), 1717-1724. (ISSN: 1546-1955; Scopus Indexed,h-index 43). [ ]
|
22.
|
ABSTRACT:
The field of image processing is addressed significantly by the role of CBIR. Peculiar query is the main feature on which the image retrieval of content based problems is dependent. Relevant information is required for the submission of sketches or drawing and similar type of features. Many algorithms are used for the extraction of features which are related to similar nature. The process can be optimized by the use of feedback from the retrieval step. Analysis of color and shape can be done by the visual contents of image. Here neural network, Relevance feedback techniques based on image retrieval are discussed.
S. Selvaraj, K. Seetharaman, (2019). Content Based Image Retrivel Based on Non-Parametric Statistical Tests of Hypothesis, International Journal of Computer Sciences and Engineering, 7(1): 563-568. (ISSN: 2347-2693 -UGC Listed in 2019).[ ]
|
23.
|
ABSTRACT:
In an automatic image retrieval system, it is difficult to understand the nature of an image data. If an automatic image retrieval system has prior knowledge about the image data, in the context of content-based image retrieval, it will give better results. The proposed method addresses this problem by applying an adaptive distance measure, Chernoff distance. The Chernoff distance adapts itself according to nature of the image data. First, the proposed method tests whether the image is a color or grayscale. If it is a color, which is converted to HSV color space; otherwise, it is treated as a grayscale. The query image is tested whether it is a structure or texture. If it is a texture, the whole image is subjected to experiment. If the image is a structure, which is segregated into various homogeneous regions, and features are extracted region-wise; and forms a feature vector (FV) region-wise. The FV of the query image is compared with the FVs in the feature vector database (FV db ) by the Chernoff distance. If the Chernoff distance is less than or equal to the critical value of the Chi-square table, then it is inferred that the query and target images are same or similar; otherwise, the images differ. The performance of the proposed method is evaluated, based on the precision and recall, and the ANMRR scores. The obtained precision and recall, and the ANMRR scores were compared with the state-of-the-art methods, which reveal that the proposed method yields better results.
K. Seetharaman, and Chembian, W. T. (2019). Color Image Retrieval Based on Adaptive Statistical Distance Measure with Local Features, International Journal of Advanced Scientific Research and Management, 4(4), 162-173. (ISSN: 2455-6378; UGC Listed in 2019). [ ]
|
24.
|
ABSTRACT:
This paper proposes a novel method, based on a probabilistic distance measure, penalized Hellinger's distance. The proposed method converts the input color query image to the HSV color space. The texture and structure components of the query imagery are segregated and compared to the target imageries in the imagery database individually at a specific significance level. If both the texture and structure components pass the test at a significance level, then it is assumed that the query imagery belongs to the same or similar class of imageries. The target imageries are marked, indexed, and retrieved. Otherwise, it is assumed that the query imagery does not match with the imageries in the imagery database. The attained results reveal that the proposed probabilistic distance-based method yields better results.
K. Seetharaman, and Chembian, W. T. (2018). Multispectral Satellite Imagery Retrieval Based on Probabilistic Distance Measure: International Journal of Research in Electronics and Computer Engineering, 6(4), 1358-1363. (ISSN: 2348-2281; UGC Listed in 2018). [ ]
|
25.
|
ABSTRACT:
The field of image processing is addressed significantly by the role of CBIR. Peculiar query is the main feature on which the image retrieval of content based problems is dependent. Relevant information is required for the submission of sketches or drawing and similar type of features. Many algorithms are used for the extraction of features which are related to similar nature. The process can be optimized by the use of feedback from the retrieval step. Analysis of color and shape can be done by the visual contents of image. Here neural network, Relevance feedback techniques based on image retrieval are discussed.
S. Selvaraj and K. Seetharaman, (2018). Color Image Retrieval Based on Chernoff Distance Measure: International Journal of Computer Sciences and Engineering, 6(9), 329-333. (ISSN: 2347-2693; UGC Listed in 2018). [ ]
|
26.
|
ABSTRACT:
This paper proposes a new method, test for equality of covariance and test for mean vectors, which retrieves different kinds of color and gray-scale images such as structured, semi-structured, textured, scaled and rotated, and noisy. The proposed method, first, performs preprocessing and then employ the test statistic, such as test for equality of covariance and generalized component test. If the query and target images pass the covariance test, then it is proceeded to test the mean vectors of the two images; otherwise, the test is dropped. If the query and target images pass both tests, then it is concluded that the images are same or similar. Otherwise, they differ. In order to validate the performance of the proposed method, an image database and feature database have been constructed. The images have been collected from various databases to maintain heterogeneity of the images. The proposed method outperforms the existing methods.
K. Seetharaman, and Gomathi,D. (2018). Content-Based Image Retrieval Based on Statistical Properties: International Journal of Data Mining and Emerging Technologies, 8(1), 58-65. (ISSN: 2249-3212). [ ]
|
27.
|
ABSTRACT:
This paper introduces a new method, based fullrange autoregressive model, for image inpainting. Themodel parameters are estimated using iterative techniqueand Bayesian methods. Based on the parameters,coefficients of the model are computed. Autocorrelationcoefficients are computed using the model coefficients.Based on the autocorrelation, the damaged region or noisedregions are identified. The identified region is inpainted thefull range autoregressive model. The performance of theproposed method is measured based on the MSE and PSNRvalues. The obtained results reveal that the proposed methodyields better results than the existing methods
K. Seetharaman, and Ramesh Babu, H. (2018). Image Inpainting Using Second-Order Derivative-Based Autoregressive Model International Journal of Electrical Electronics & Computer Science Engineering, 5(1), 24-28. (ISSN: 2454-1222). [ ]
|
28.
|
ABSTRACT:
Object recognition is presently one of the most active research areas in computer vision, pattern recognition, artificial intelligence andhuman activity analysis. The area of object detection and classification, attention habitually focuses on changes in the location of anobject withrespect to time, since appearance information can sensibly describe the object category. In this paper, feature set obtained from the Gray LevelCo-Occurrence Matrices (GLCM), representing a different stage of statistical variations of object category. The experiments are carried outusing Caltech 101 dataset, considering sevenobjectsviz (airplanes, camera, chair, elephant, laptop, motorbike and bonsai tree) and the extractedGLCM feature set are modeled by tree based classifier like Naive Bayes Tree and Random Forest. In the experimental results, Random Forestclassifier exhibits the accuracy and effectiveness of the proposed method with an overall accuracy rate of 89.62%, which outperforms the Naďve Bayes classifier.
Gomathi, D. and K. Seetharaman, (2018). Object Classification Techniques using Tree Based Classifiers: International Journal on Future Revolution in Computer Science & Communication Engineering,4(1), 271-276. (ISSN: 2454-4248).
[ ]
|
29.
ABSTRACT:
This paper proposes a novel method based on statistical tests of hypotheses, such as F-ratio and Welch's t-tests. The input query image is examined whether it is a textured or structured. If it is structured, the shapes are segregated into various regions according to its nature; otherwise, it is treated as textured image and considered the entire image as it is for the experiment. The aforesaid tests are applied regions-wise. First, the F-ratio test is applied, if the images pass the test, then it is proceeded to test the spectrum of energy, i.e. means of the two images. If the images pass both tests, then it is concluded that the two images are the same or similar. Otherwise, they differ. Since the proposed system is distribution-based, it is invariant for rotation and scaling. Also, the system facilitates the user to fix the number of images to be retrieved, because the user can fix the level of significance according to their requirements. These are the main advantages of the proposed system.
|
K. Seetharaman, and S. Selvaraj, (2016) Statistical Tests of Hypothesis-Based Color Image Retrieval, Journal of Data Analysis and Information Processing, Vol. 4(2), pp. 90-99, May 2016 (ISSN: 2327-7211; Scopus indexed. [ ]
|
30.
ABSTRACT:
A Framework for track human motion in an enclosed atmosphere from sequences of monocular gray scale pictures that are obtained from mounted cameras. The detection of objects that are moving uses background subtraction algorithm which is working based on Gaussian mixture models. Variable Gaussian models square measure applied to seek out the most likely matches of human movements between successive frames taken by cameras mounted in varied locations. The modified Kalman filters is used for tracking objects in each frame, and determine the possibility of each detection is being assigned to each track. An important aspect of this project is Track maintenance.
|
K. Seetharaman, N.Palanivel, and M.Vasanthapriya, (2016) An Efficient Real Time People Counting System Based on Identification and Tracking Using Surveillance Camera, International Journal of Advanced Research in Computer Science Engineering and Information Technology, Vol. 4(3), pp. 495-501, Mar,2016, (ISSN: 2321-3337). [ ]
|
31.
ABSTRACT:
This paper proposes a novel method, based on a statistical probability distributional approach, Hotelling’s T2 statistic and Orthogonality test. If the input query image is structured, it is segmented into various regions according to its nature and structure. Otherwise, the image is treated as textured; and it is considered for the experiment as it is. The test statistic T2 is applied on each region and compares it to the target image. If the test of hypothesis is accepted, it is inferred that the query and target images are same or similar. Otherwise, it is assumed that they belong to different groups. Moreover, the Eigen vectors are computed on each region, and the orthogonality test is employed to measure the angle between the two images. The obtained results outperform the existing methods.
|
K. Seetharaman, and S. Muthukumar, (2016) A Distributional Approach for Image Retrieval Using Hotelling’s T-Square Statistic, ICTACT Journal on Image and Video Processing, Vol. 6(3), pp. 1207-1212, February, 2016(ISSN: 0976 9099). [ ]
|
32.
ABSTRACT:
This paper proposes a novel technique, based on Canonical correlation analysis, and the Chi-square test is employed to test the significance of the correlation coefficients. If it is significant, then it is concluded that the input query and target images are same or similar; otherwise, it is inferred that the two images are significantly different. In order to experiment the proposed canonical correlation method, a database is designed and constructed with the help of different types of images and their feature vectors. The Fβ-measure is applied to evaluate the performance of the proposed method. The obtained results expose that the proposed technique yields better results than the existing.
|
K. Seetharaman, and Bachala Shyam Kumar, (2016) Texture and Color Features Based Color Image Retrieval using Canonical Correlation, Global Journal of Researches and Engineering, Vol. 15(6), pp. 1-9, 2015, (ISSN: 0975-5861). [ ]
|
33.
ABSTRACT:
This paper presents a unified learning framework for heterogeneous medical image retrieval based on a Full Range Autoregressive Model (FRAR) with the Bayesian approach (BA). Using the unified framework, the color autocorrelogram, edge orientation autocorrelogram (EOAC) and micro-texture information of medical images are extracted. The EOAC is constructed in HSV color space, to circumvent the loss of edges due to spectral and chromatic variations. The proposed system employed adaptive binary tree based support vector machine (ABTSVM) for efficient and fast classification of medical images in feature vector space. The Manhattan distance measure of order one is used in the proposed system to perform a similarity measure in the classified and indexed feature vector space. The precision and recall (PR) method is used as a measure of performance in the proposed system. Short-term based relevance feedback (RF) mechanism is also adopted to reduce the semantic gap. The Experimental results reveal that the retrieval performance of the proposed system for heterogeneous medical image database is better than the existing systems at low computational and storage cost.
|
K. Seetharaman and S. Sathiamoorthy, (2016) A Unified Learning Framework for Content Based Medical Image Retrieval Using a Statistical Model, Journal of King Saud University - Computer and Information Sciences (Article in press – Elsevier, ISSN: 1319-1578). [ ]
|
34.
ABSTRACT:
In this paper, a data hiding scheme with distortion tolerance for videos is proposed, which is generally used to embed secret information into the cover video for secure transmission and protecting copyright. The secret information feasibly is a text or an image. To protect the copyright of a video, a signature represented by a sequence of binary data is embedded into the video. In the proposed scheme, initially the embedding error between the frame of the cover video and the secret information is calculated. Based on this embedding error, the stego-video is computed then the embedded data are extracted by the extraction procedure. This scheme can tolerate some distortion such as salt and pepper noise, Gaussian noise, and uniform noise, when transmitting a stego-video through any network. Experimental results and discussions reveal that the proposed scheme tolerates those distortions with acceptable video quality.
|
R. Ragupathy, K. Seetharaman, and M. Saveetha. (2015). Embedding Error Based Data Hiding in Videos with Distortion Tolerance, , International Journal of Applied Engineering Research, 10(8), pp. 6186-6190. (ISSN: 0973-4562; Scopus Indexed ). [ ]
|
35.
ABSTRACT:
This paper proposes a novel technique, based on Canonical correlation analysis, and the Chi-square test is employed to test the significance of the correlation coefficients. If it is significant, then it is concluded that the input query and target images are same or similar; otherwise, it is inferred that the two images are significantly different. In order to experiment the proposed canonical correlation method, a database is designed and constructed with the help of different types of images and their feature vectors. The Fβ-measure is applied to evaluate the performance of the proposed method. The obtained results expose that the proposed technique yields better results than the existing.
|
K. Seetharaman, and Shyam Kumar, (2015) Colour Image Retrieval Using Canonical Correlation Analysis, Annamalai University Science Journal, Vol. 49(2), pp. 99-104, October 2015 (ISSN: 2231 0827). [ ]
|
36.
ABSTRACT:
This paper proposes a novel technique based on feature fusion using multiple linear regression analysis, and the least-square estimation method is employed to estimate the parameters. The given input query image is segmented into various regions according to the structure of the image. The color and texture features are extracted on each region of the query image, and the features are fused together using the multiple linear regression model. The estimated parameters of the model, which is modeled based on the features, are formed as a vector called a feature vector. The Canberra distance measure is adopted to compare the feature vectors of the query and target images. The Fmeasure is applied to evaluate the performance of the proposed technique. The obtained results expose that the proposed technique is comparable to the other existing techniques.
|
K. Seetharaman, and R. Shekhar, (2015) Color Image Retrieval Based On Feature Fusion Through Multiple Linear Regression Analysis, ICTACT Journal on Image and Video Processing , August 2015, Vol. 6(1), pp. 1666-1071.(ISSN: 0976-9099). [ ]
|
37.
ABSTRACT:
The field of image processing is addressed significantly by the role of CBIR. Peculiar query is the main feature on which the image retrieval of content based problems is dependent. Relevant information is required for the submission of sketches or drawing and similar type of features. Many algorithms are used for the extraction of features which are related to similar nature. The process can be optimized by the use of feedback from the retrieval step. Analysis of color and shape can be done by the visual contents of image. Here neural network, Relevance feedback techniques based on image retrieval are discussed.
|
S. Selvaraj and K. Seetharaman, (2015) CBIR Based on Non-Parametric Statistical Tests of Hypothesis, , Australian Journal of Basic and Applied Sciences, Vol. 9(11) May, 2015, pp. 322-328 (ISSN: 1991-8178 ; Scopus indexed ) [ ]
|
38.
|
ABSTRACT:
This paper proposes a novel system, based on Full Range Gaussian Markov Random Field (FRGMRF) model with Bayesian approach for image retrieval. The color image is segmented into various regions according to its structure and nature. The segmented image is modeled to HSV color space, where V attributes to pixel values, and it ranges from 0 to 1. On HSV color space, the texture information and spatial orientation of the pixels are extracted. On each region, the model parameters, autocorrelation coefficient (ACC), and unique texture numbers are computed using the FRGMRF model. The model parameters and ACCs are formed as feature vectors (FVs) of the image. The Mahalanobis distance is applied to measure the distance between the query and target images. Moreover, associated probabilities are computed on the texture numbers on each region, and are used to compute the divergence between the query and target images using cosine function. The obtained results are compared to those of the existing systems. The comparative study reveals that the proposed system outperforms the existing systems.
K. Seetharaman, (2015) Image Retrieval Based on Micro-level Spatial Structure Features and Content Analysis Using Full Range Gaussian Markov Random Field Model, Engineering Applications of Artificial Intelligence, Vol. 40, pp. 103-116, April, 2015 (Elsevier – DOI: 10.1016/j.engappai. 2015.01.008; ISSN: 0952-1976; Scopus indexed; Impact Factor: 3.526 ).
[ ]
|
39.
|
ABSTRACT:
This paper proposes a novel system, based on Full Range Gaussian Markov Random Field (FRGMRF) model with Bayesian approach for image retrieval. The color image is segmented into various regions according to its structure and nature. The segmented image is modeled to HSV color space, where V attributes to pixel values, and it ranges from 0 to 1. On HSV color space, the texture information and spatial orientation of the pixels are extracted. On each region, the model parameters, autocorrelation coefficient (ACC), and unique texture numbers are computed using the FRGMRF model. The model parameters and ACCs are formed as feature vectors (FVs) of the image. The Mahalanobis distance is applied to measure the distance between the query and target images. Moreover, associated probabilities are computed on the texture numbers on each region, and are used to compute the divergence between the query and target images using cosine function. The obtained results are compared to those of the existing systems. The comparative study reveals that the proposed system outperforms the existing systems.
Thillaikarasi and K. Seetharaman, (2015) Efficiency of Test Case Prioritization Techniques Based on Practical Priority Factors: International Journal of Soft Computing, 10(2), 183-188.(ISSN: 1816-9503; Scopus indexed) ). [ ]
|
40.
ABSTRACT:
This paper proposes a novel method, based on Full Range Autoregressive (FRAR) model with Bayesian approach for color image retrieval. The color image is segmented into various regions according to its structure and nature. The segmented image is modeled to RGB color space. On each region, the model parameters are computed. The model parameters are formed as a feature vector of the image. The Hotlling T2 Statistic distance is applied to measure the distance between the query and target images. Moreover, the obtained results are compared to that of the existing methods, which reveals that the proposed method outperforms the existing methods.
Keywords- FRAR model, query image, target image, feature vector, spatial features.
|
AnnamalaiGiri and K. Seetharaman, (2014) Color Image Retrieval Based on Full Range Color Image Retrieval Based on Full Range Gaussian Markov Random Field Model, International Journal of Innovative Research in Advanced Engineering, Vol. 1(1), pp. 62-70 (ISSN: 2349-2163; Impact Factor: 1.013). [ ]
|
41.
ABSTRACT:
A novel method for color image retrieval based on statistical non-parametric tests such as two-sample Wald Test for equality of variance and Man-Whitney U test is proposed in this paper. The proposed method tests the deviation, i.e. distance in terms of variance between the query and target images; if the images pass the test, then it is proceeded to test the spectrum of energy, i.e. distance between the mean values of the two images; otherwise, the test is dropped. If the query and target images pass the tests then it is inferred that the two images belong to the same class, i.e. both the images are same; otherwise, it is assumed that the images belong to different classes, i.e. both images are different. The obtained test statistic values are indexed in ascending order and the image corresponds to the least value is identified as same or similar images. Here, either the query image or the target image is treated as sample; the other is treated as population. Also, some other features such as Coefficient of Variation, Skewness, Kurtosis, Variance, and Spectrum of Energy are compared between the query and target images color-wise. The proposed method is robust for scaling and rotation, since it adjusts itself and treats either the query image or the target image is the sample of other. The results obtained are comparable with the existing methods.
Keywords-Variance, mean, query image, target image, non-parametric tests.
|
K. Seetharaman and M. Jeyakarthic, (2014) Color Image Retrieval Based on Non-parametric Statistical Tests of Hypothesis, International Journal of Emerging Technology and Advanced Engineering, Vol. 4(8), pp. 750-756. (ISSN: 2250-2459). [ ]
|
42.
ABSTRACT:
This paper proposes wavelet based orthogonal polynomial coefficients model for content based image retrieval (CBIR). The coefficients are categorized into low-frequency and high-frequency based on a criteria. The criteria is adaptively determined and fixed according to the nature and structure of the image, because the wavelet based orthogonal polynomial model spatially localizes the frequency information. Wavelet packet and Daubechies-4 transforms are jointly used to construct both approximation (low-frequency) and detailed (high-frequency) multiresolution image subbands. Color feature are extracted from low-frequency subband based on color autocorrelogram, whereas texture features are extracted from high-frequency subband based on co-occurrence matrix. Based on these features, the feature vector is formed. The proposed CBIR method reduces the feature variation when different modalities of images are combined. The proposed system assessed two medical image databases and one general image database with Minkowski-form distance method. The experimental results show that the proposed method achieves comparable retrieval performance with medical dataset; moreover, it is very fast with low computational load. Further, the obtained results were compared with other recently developed methods such as highly adaptive wavelet method, Wavelet optimization method and effective CBIR techniques. The proposed method yields better results compared to that of existing methods.
|
K. Seetharaman and M. Kamarasan, (2014) Statistical Framework for Content-Based Medical Image Retrieval Based on Orthogonal Polynomial with Multiresolution Structure, International Journal of Multimedia Information Retrieval, Vol. 3(1), pp. 53-66, 2014 (Springer – DOI: 10.100/s13735-013-0048-2; ISSN: 2192-6611; Scopus indexed; Impact Factor: 0.63 ).
[ ]
|
43.
ABSTRACT:
A content based image retrieval (CBIR) system for diverse collection of color images is proposed. The proposed image retrieval framework consists of Full Range Gaussian Markov Random Field (FRGMRF) model with Bayesian Approach (BA) for image feature extraction and multi-class Support Vector Machine (SVM) learning mechanism for the categorization of image feature vectors in the feature vector database. The Minkowski distance based similarity measure is performed in the final level on the pre-filtered images. In order to incorporate a better perception subjectivity, relevance feedback (RF) technique is added to update the query image dynamically. Experiments are conducted on various image databases. The experimental results based on precision and recall method are reported. Experimental result demonstrates the progress, effectiveness, and efficiency achieved by the proposed framework. Keywords- Content based image retrieval, Full Range Gaussian Markov Random Field model, Support vector machine, Relevance feedback, Bayesian approach.
|
K. Seetharaman and S. Sathiamoorthy, (2014) A Framework for Color Image Retrieval Using Full Range Gaussian Markov Random Field Model and Multi-class SVM Learning Approach, International Journal Advanced Research in Engineering, Vol. 1(7), pp. 53-63 (ISSN: 2349-2163). [ ]
|
44.
ABSTRACT:
This paper proposes a new and effective framework for color image retrieval based on Full Range Autoregressive Model (FRAR). Bayesian approach (BA) is used to estimate the parameters of the FRAR model. The color autocorrelogram, a new version of edge histogram descriptor (EHD) and micro-texture (MT) features are extracted using a common framework based on the FRAR model with BA. The extracted features are combined to form a feature vector, which is normalized and stored in image feature vector database. The feature vector database is categorized according to the nature of the images using the radial basis function neural network (RBFNN) and k-means clustering algorithm. The proposed system adopted Manhattan distance measure of order one to measure the similarity between the query and target images in the categorized and indexed feature vector database. The query refinement approach of short-term learning based relevance feedback mechanism is adopted to reduce the semantic gap. The experimental results, based on precision and recall method are reported. It demonstrates the performance of the improved EHD, effectiveness and efficiency achieved by the proposed framework..
|
K. Seetharaman and S. Sathiamoorthy, (2014) Color Image Retrieval Using Statistical Model and Radial Basis Function Neural Network, Egyptian Informatics Journal, Vol.15(1), pp. 59-68, 2014 (Elsevier - DOI: 10.1016/j.eij.2014.02.001, ISSN: 1110-8665; Scopus Indexed; Impact Factor: 2.306;h-index 22).
[]
|
45.
ABSTRACT:
Regression testing concentrates on finding defects after a major code change has occurred. Specifically, it
exposes software regressions or old bugs that have reappeared. It is an expensive testing process that has
been estimated to account for almost half of the cost of software maintenance. To improve the regression
testing process, test case prioritization techniques organizes the execution level of test cases. Further, it
gives an improved rate of fault identification, when test suites cannot run to completion.
|
Thillaikarasi, M., K. Seetharaman, K., (2014) Effectiveness of Test Case Prioritization Techniques Based on Regression Testing, International Journal of Software Engineering & Applications, 5(6), pp. 113-123; DOI : 10.5121/ijsea.2014.5608. []
|
46.
ABSTRACT:
Regression Testing is the process of executing the set of test cases which have passed on the previous build or release of the application under test in order to validate that the original features and functions are still working as they were previously. It is impracticable and in-sufficient resources to re-execute every test case for a program if changes occur. This problem of regression testing can be solved by prioritizing test cases. A regression test case prioritization technique involves re-ordering the execution of test suite to increase the rate of fault detection in earlier stages of testing process. In this paper, test case prioritization algorithm is proposed to identify the severe faults and improve the rate of fault detection. This proposed test case prioritization algorithm prioritizes the test cases based on four groups of practical weight factor such as: customer allotted priority, developer observed code execution complexity, changes in requirements, fault impact, completeness and traceability. The proposed prioritization technique is validated with three different validation metrics and is experimented using two projects. The effectiveness of proposed technique is achieved by comparing it with un-prioritized ones and by validation metrics.
|
Thillaikarasi, M., K. Seetharaman, K., (2014) Comparisons of Test t Case Prioritization Algorithm with Random Prioritization, International Journal of Computer Science and Information Technologies, Vol. 5 (5) , 2014, pp. 6814-6818 (Scientific Research; ISSN: 0975-9646; Impact Factor:2.93). []
|
47.
ABSTRACT:
The techniques of test case prioritization schedule the execution order of test cases to attain respective target, such as enhanced level of forecasting the fault. The requirement of the prioritization can be viewed as the en-route for deriving an order of relation on a given set of test cases which results from regression testing. Alteration of programs between the versions can cause more test cases which may respond differently to following versions of software. In this, a fixed approach to prioritizing test cases avoids the preceding drawbacks. The JUnit test case prioritization techniques operating in the absence of coverage information, differs from existing dynamic coverage-based test case prioritization techniques. Further, the prioritization test cases relying on coverage information were projected from fixed structures relatively other than gathered instrumentation and execution.
Keywords:
Software Testing, Regression Testing, Test Case, Prioritization, JUnit, Call Graph
|
Thillaikarasi, M., Seetharaman, K., (2014) Regression Testing in Developer Environment for Absence of Code Coverage, Journal of Software Engineering and Applications, July, 2014, Vol. 7(8), pp. 617-625 (ISSN: 1945-3116; Impact Factor: 0.88) DOI: 10.4236/jsea.2014.78057. [ ]
|
48.
ABSTRACT:
Abstract Regression Testing is the process of executing the set of test cases which have passed on the previous build or release of the application under test in order to validate that the original features and functions are still working as they were previously. It is impracticable and in-sufficient resources to re-execute every test case for a program if changes occur. This problem of regression testing can be solved by prioritizing test cases. A regression test case prioritization technique involves re-ordering the execution of test suite to increase the rate of fault detection in earlier stages of testing process. In this paper, test case prioritization algorithm is proposed to identify the severe faults and improve the rate of fault detection. This proposed test case prioritization algorithm prioritizes the test cases based on four groups of practical weight factor such as: customer allotted priority, developer observed code execution complexity, changes in requirements, fault impact, completeness and traceability. The proposed prioritization technique is validated with three different validation metrics and is experimented using two projects. The effectiveness of proposed technique is achieved by comparing it with unprioritized ones and by validation metrics. Keywords Regression Testing, Test case prioritization, Fault severity, Rate of fault detection.
|
Thillaikarasi, M., Seetharaman, K., (2014) A New Effective Test Case Prioritization for Regression Testing based on Prioritization Algorithm, International Journal of Applied Information Systems, Vol.6(7), January, 2014, pp. 21-26 (ISSN: 22490868). [ ]
|
49.
ABSTRACT:
Dynamic texture is the texture which is in motion. Dynamic texture segmentation is a challenging task, as the texture can change in shape and direction over time. In this paper segmentation of Dynamic textures into distinct regions has been done. For the segmentation of dynamic texture, three different techniques are combined together to obtain better segmentation. Two local texture descriptors called Local binary pattern and Weber local descriptor are used. These descriptors, when used in spatial domain helps to segment a frame of video into distinct regions based on the histogram of the region. Also using the same texture descriptors in temporal domain, it is possible to obtain the dynamic texture in a given video. In addition to these texture descriptors, optical flow of pixels is used for detecting dynamic texture in a video, as optical flow is the natural method for detection of motion. After computing the three different features from multiple split sections of a group of video frames, individual histograms are obtained for each of the split sections of the video. These histograms each are converted to a single value called the Cumulative. The cumulative so obtained is compared with the threshold and filtered to obtain the dynamic texture. Since the histograms are converted to a single value, the computation of threshold is very easy as the whole set of values of Cumulative falls in two different set of values. The magnitude of motion detected depends on the threshold selected.
Keywords- Dynamic texture- Dynamic texture, segmentation, Cumulative, Texture descriptor, optical flow.
|
K. Seetharaman, N. Palanivel and D. Sowmya, (2014) Video Segmentation in Dynamic Texture Using Weber’s Law for Monitoring Different Environments, International Journal of Innovative Science, Engineering and Technology, vol. 1(2), pp. 230-234 (ISSN: 2348-7968).
[ ]
|
50.
ABSTRACT:
An embedded system is presented in which face recognition and facial recognition for Human-Robot Interaction are implemented. To detect face with a fast and reliable way, HMM combined with SVM algorithm is used. The full pose from face recognition data base is considered to detect the face recognition. Performance of the face recognition reaches to 99.617%. The two main advantages of our method are that it does not require manually selected facial landmarks or head pose estimation. In order to improve the performance of our pose normalization method in face recognition, an algorithm is presented for classifying whether a given face image is at a frontal or non frontal pose. In addition to the proposed method, a pre processing state of detecting the face is included here. The images which are non faces are detected and eliminated from the database is an another main advantage in of this work.
|
K. Seetharaman, N. Palanivel and R. Indumathi, (2014) Post Invariant Face Recognition Using HMM and SVM with PCA for Dimensionality Reduction, International Journal of Advanced and Innovative Research, Vol. 3(1), pp. 171-176 (ISSN: 2278-7844).
[ ]
|
51.
ABSTRACT:
Image degradation usually occurs when certain image losses the stored information due to conversion or digitalization that decreases the quality of the image. Variation
in brightness of color information in images is usually called as noise. Image usually subjects to
noise of many types. Gaussian noises that occur in the image are concentrated. The principle of
Fourier transformation is used so as to decompose the image into sine and cosine components for
representing the image in particular frequency. Image histograms are an important technique for
inspecting images that are used to spot the background and grey value range at a glance. The
concepts of spatiogram with both first order and second order are to enhance the image with high
definition clarity thereby removing the degradation in image. In addition, the images were preevaluated before image manipulation process.
Keywords: Gaussian noise, Fourier transformation, Histogram, Spatiogram
|
K. Seetharaman, N. Palanivel and K. Maruthavanan, (2014) Histogram validated object removal strategy using spatiogramic technique, International Journal of Advanced Research in Computer Science Engineering and Information Technology, vol. 4(4), pp. 1-7 (ISSN: 2347-9817). [ ]
|
52.
ABSTRACT:
This paper proposes a unified framework for color image retrieval, based on statistical multivariate parametric tests, namely test for equality of covariance matrices, test for equality of mean vectors, and the orthogonality test. The proposed method tests the variation between the query and target images; if it passes the test, then it proceeds to test the spectrum of energy of the two images; otherwise, the test is dropped. If the query and target images pass both the tests then it is concluded that the two images belong to the same class, i.e. both the images are same; otherwise, it is assumed that the images belong to different classes, i.e. both the images are different. The obtained test statistic values are indexed in ascending order and the image corresponds to the least value is identified as same or similar images. Here, either the query image or target image is treated as sample; the other is treated as population. Also, some other features such as Coefficient of Variation, Skewness, Kurtosis, Variance-Covariance, Spectrum of Energy, and number of shapes in the images are compared between the query and target images color-wise. Furthermore, to emphasize the efficiency of the proposed system, the geometrical structure, viz. test for orthogonality between the query and target images, is examined. In the case of structure images, the number of shapes in the query and target images are compared; if it matches, then the contents in the shapes are compared color wise. The proposed system is invariant for scaling, and rotation, since the proposed system adjusts itself and treats either the query image or the target image is the sample of other. The proposed framework provides hundred percent accuracy if the query and target images are same, whereas there is a slight variation for similar, scaled, and rotated images.
|
K. Seetharaman and M. Jeyakarthic, (2014) Statistical Distributional Approach for Scale and Rotation Invariant Color Image Retrieval Using Multivariate Parametric tests and Orthogonality Condition, Journal of Visual Communication and Image Representation, Vol. 25(5), pp. 727-739, 2013 (Elsevier – DOI: 10.1016/j.jvcir.2014.01.004; ISSN: 1047-3203; Scopus indexed; Impact Factor: 2.259 ).
[ ]
|
53.
ABSTRACT:
The advent of large scale digital image database leads to great challenges in contentbased image retrieval (CBIR) method. The CBIR is considered an active area of research; however, it comprises a strong backdrop for new methodologies and system implementations. Hence, many research contributions focus on these techniques to enable higher image retrieval accuracy while preserving the low level computational complexity. This paper proposes a CBIR method, which is based on an efficient combination of multiresolution based color and texture features. This paper considers color autocorrelogram of the hue(H) and saturation(s) components of HSV color space for color features, and value(V) component of HSV color space for texture features. These two image features are extracted by computing co-occurrence matrix at optimum level image, which is the basis for the formation of feature vector. Though the optimum level is constructed based on wavelet transform, which contains a few dominant wavelet coefficients. The efficiency of the proposed system is tested with standard image databases, and the experimental results show that the proposed method achieves better retrieval accuracy at optimum level; moreover, the proposed method is very fast with low computational load. The obtained results are compared with existing techniques such as orthogonal polynomial model, multiresolution with BDIP-BVLC method and GLCMbased system, and results reveal that the proposed method outperforms the existing methods.
|
K. Seetharaman and M. Kamarasan, (2014)Statistical Framework for Image Retrieval Based on Multiresolution Features and Similarity Method, Multimedia Tools and Applications, Vol. 73(3), pp. 1943-1962, 2014 (Springer – DOI: 10.1007/s11042-013-1637-z; ISSN: 1380-7501; Scopus indexed; Impact Factor:2.101).
[ ]
|
54.
ABSTRACT:
Scheduling test cases by using test case prioritization technique enhances their efficiency of attaining some performance criteria. The rate at which the errors are detected within the testing process is one such criteria. An enhanced rate of fault detection during testing can provide quicker feedback on the system under test thereby allowing s/w engineers to rectify errors before usual time. An application of prioritization techniques includes regression testing of s/w which has undergone alterations. To optimize regression testing s/w testers may assign test case preferences so that to some extent the more significant ones are run earlier in the regression testing process. Software testing and retesting is an inherent part of software development as far as the errors are detected at the earliest that may not change the sequence hence gaining confidence. Regression testing has been proved to be crucial stage of software testing .Regression test prioritization techniques manipulates the execution of test case so that faults are detected at the earliest. To achieve performance requirements test cases with higher priority are executed than those with lower priority by test case prioritization techniques. This proposed test case prioritization algorithm prioritizes the test cases based on four groups of practical weight factors such as Time factor, Defect factors, Requirement factor and complexity factors. The proposed technique is validated with three different validation metrics and is experimented using two projects The algorithm illustrated detects serious errors at earlier phases of testing process and effectiveness between prioritized and un prioritized test cases is compared using ASFD. KEY WORDS: Regression Testing, Test case, Test case prioritization, Fault severity, Rate of fault detection
|
Thillaikarasi, M., Seetharaman, K., [2013]A Test Case Prioritization Method with Weight Factors in Regression Testing Based on Measurement Metrics, International Journal of Advanced Research in Computer Science and Software Engineering, December, 2013, Vol. 3(12), pp. 390-396 (ISSN: 2277-128X). [ ]
|
55.
ABSTRACT:
A statistical approach, based on full range Gaussian Markov random field model, is proposed for texture analysis such as texture characterization, unique representation, description, and classification. The parameters of the model are estimated based on the Bayesian approach. The estimated parameters are utilized to compute autocorrelation coefficients. The computed autocorrelation coefficients fall in between -1 and +1. The coefficients are converted into decimal numbers using a simple transformation. Based on the decimal numbers, two texture descriptors are proposed: (i) texnum, the local descriptor; (ii) texspectrum, the global descriptor. The decimal numbers are proposed to represent the textures present in a small image region. These numbers uniquely represent the texture primitives. The textured image under analysis is represented globally by observing the frequency of occurrences of the texnums called texspectrum. The textures are identified and are distinguished from untextured regions with edges. The classification analyses such as supervised and unsupervised are performed on the local descriptors.
|
K. Seetharaman and N. Palanivel, (2013) Texture Characterization, Representation, Description and Classification Based on a Family of Full Range Gaussian Markov Random Field Model, International Journal of Image and Data Fusion, Vol. 4(4), 2013, pp. 342-362 (Taylor and Francis, ISSN: 1947-9832; Scopus indexed ).
[ ]
|
56.
ABSTRACT:
The advanced applications and services to incorporate the context awareness are required by the persisting advances in the fields of wireless network and mobile computing. In distributed mobile networks, context awareness is considered as a vital and beneficial feature. Generally, mobile devices have certain constraints such as processing, storage space etc which can be overcome by using context awareness. Context plays a major role in filtering the data and services transmitted to the devices which results in reduced processing cost. In this paper, we design ontology based middleware architecture for context aware service discovery. The architecture uses contextual ontologies to allow the semantically enhanced contextual requests for services.
|
Christopher Siddarth and K. Seetharaman, (2013) Ontology Based Middleware Architecture for Context Aware Service Discovery, European Journal of Scientific Research, Vol. 101(2), 2013, pp. 303-317, (ISSN: 1450-216X/1450-202X; Impact Factor: 0.713; Scopus indexed). [ ]
|
57.
ABSTRACT:
The existing mobile service discovery approaches do not completely address the issues of service selection and the robustness faced to mobility. The infrastructure of mobile service must be QoS-aware plus context-aware (i.e.) aware of the user's required-QoS and the QoS offered by the other networks in user's context. In this paper, we propose a cluster based QoS-aware service discovery architecture using swarm intelligence. Initially, in this architecture, the client sends a service request together with its required QoS parameters like power, distance, CPU speed etc. to its source cluster head. Swarm intelligence is used to establish the intra and inter cluster shortest path routing. Each cluster head searches the QoS aware server with matching QoS constraints by means of a service table and a server table. The QoS aware server is selected to process the service request and to send the reply back to the client. By simulation results, we show that the proposed architecture can attain a good success rate with reduced delay and energy consumption, since it satisfies the QoS constraints.
KEYWORDS:
QoS-Aware, Ant Colony Optimization (ACO), Swarm Intelligence, Mobile Ad Hoc Networks (MANETs)
|
Christopher Siddarth and K. Seetharaman, (2013) A Cluster Basesd QoS - Aware Service Discovery Architecture Using Swarm Intelligence, Communications and Network, Vol. 5(2), 2013, pp. 161 – 168,(Scientific Research, ISSN: 1949-2421; Impact Factor: 0.37). [ ]
|
58.
ABSTRACT:
This paper proposes a Full Range Gaussian Markov Random Field (FRGMRF) model for monochrome image compression, where images are assumed to be Gaussian Markov Random Field. The parameters of the model are estimated based on Bayesian approach. The advantage of the proposed model is that it adapts itself according to the nature of the data (image) because it has infinite structure with a finite number of parameters, and so completely avoids the problem of order determination. The proposed model is fitted to reconstruct the image with the use of estimated parameters and seed values. The residual image is computed from the original and the reconstructed images. The proposed FRGMRF model is redefined as an error model to compress the residual image to obtain better quality of the reconstructed image. The parameters of the error model are estimated by employing the Metropolis-Hastings (M-H) algorithm. Then, the error model is fitted to reconstruct the compressed residual image. The Arithmetic coding is employed on seed values, average of the residuals and the model coefficients of both the input and residual images to achieve higher compression ratio. Different types of textured and structured images are considered for experiment to illustrate the efficiency of the proposed model. The results obtained by the FRGMRF model are compared to the JPEG2000. The proposed approach yields higher compression ratio than the JPEG whereas it produces Peak Signal to Noise Ratio (PSNR) with little higher than the JPEG, which is negligible.
Keywords: Image Compression; FRGMRF Model; Bayesian Approach; Seed Values; Error Model
|
K. Seetharaman and V. Rekha,(2013) Near-Lossless Compression Based on A Family of Full Range Autoregressive Model for 2D Monochrome Images, Journal of Signal and Information Processing, Vol.4(1), 2013, pp. 10 – 23, (Scientific Research, ISSN: 2159-4465). [ ]
|
59.
ABSTRACT:
This paper introduces a novel approach, i.e. block oriented-restoration, based on a Family of Full Range Autoregressive (FRAR) model to restore the information lost, and this adopts the Bayesian approach to estimate the parameters of the model. The Bayesian approach, by combining the prior information and the observed data known as posterior distribution, makes inferences. The loss of information caused is due to errors in communication channels, through which the data are transmitted. In most applications, the data are transmitted block wise. Even if there is loss of a single bit in a block, it causes loss in the whole block and the impact may reflect on its consecutive blocks. In the proposed technique, such damaged blocks are identified, and to restore it, a priori information is searched and extracted from uncorrupted regions of the image; this information and the pixels in the neighboring region of the damaged block are utilized to estimate the parameters of the model. The estimated parameters are employed to restore the damaged block. The proposed algorithm takes advantage of linear dependency of the neighboring pixels of the damaged block and takes them as source to predict the pixels of the damaged block. The restoration is performed at two stages: first, the lone blocks are restored; second, the contiguous blocks are restored. It produces very good results and is comparable with other existing schemes.
|
K. Seetharaman,(2012) A Block-oriented Restoration in Gray-scale Images Using Full Range Autoregressive Model, Pattern Recognition, Vol. 45(4), April, 2012, pp. 1591-1601 (Elsevier, ISSN: 0031-3203; Scopus indexed; Impact Factor:5.898 ).
[ ]
|
50.
ABSTRACT:
We use Low Density Parity Check (LDPC) error correction code to solve fuzziness i. e. , the variability and noise in iris code generated from Iris Recognition System (IRS) and Secure Hash Algorithm (SHA-512) to transform unique iris code into hash string to make them as a Cancellable Biometric. SHA-512 hash string is used as a key for 512 bits Advanced Encryption Standard (AES) encryption process. In the decryption process, Hash comparison is made between new hash generated by SHA-512 from error corrected iris code and hash stored in smart card. If authentic, 512 bits AES decryption is accomplished. Experiments indicate that, use of LDPC code results in better separation between genuine and impostor users which improves the performance of this novel cryptosystem. Security of this system is very high, which is 2256 under birthday attack. AES algorithm is enhanced by using 512 bits key and increasing the number of rounds.
|
K. Seetharaman and R. Ragupathy, (2012) A Novel Biometric Cryptosystem using LDPC and SHA based Iris Recognition, International Journal of Applied Information Systems, Vol. 4(1), pp. 41 – 47, 2012. (ISSN: 2249-0868). [ ]
|
61.
ABSTRACT:
We introduce a novel way to authenticate an image using Low Density Parity Check (LDPC) and Secure Hash Algorithm (SHA) based iris recognition method with reversible watermarking scheme, which is based on Integer Wavelet Transform (IWT) and threshold embedding technique. The parity checks and parity matrix of LDPC encoding and cancellable biometrics i.e., hash string of unique iris code from SHA-512 are embedded into an image for authentication purpose using reversible watermarking scheme based on IWT and threshold embedding technique. Simply by reversing the embedding process, the original image, parity checks, parity matrix and SHA-512 hash are extracted back from watermarked-image. For authentication, the new hash string produced by employing SHA-512 on error corrected iris code from live person is compared with hash string extracted from watermarked-image. The LDPC code reduces the hamming distance for genuine comparisons by a larger amount than for the impostor comparisons. This results in better separation between genuine and impostor users which improves the authentication performance. Security of this scheme is very high due to the security complexity of SHA-512, which is 2256 under birthday attack. Experimental results show that this approach can assure more accurate authentication with a low false rejection or false acceptance rate and outperforms the prior arts in terms of PSNR.
|
K. Seetharaman and R. Ragupathy, (2012) LDPC and SHA based iris recognition for image authentication, Egyptian Informatics Journal, Vol. 13(3), pp. 217 – 224, 2012 (Elsevier ISSN: 1110-8665; Scopus indexed; Impact Factor:2.306 ).
[ ]
|
62.
ABSTRACT:
This paper introduces an efficient approach to protect the ownership by hiding iris code from iris recognition system into digital image for an authentication purpose using the reversible watermarking scheme. This scheme embeds bookkeeping data of histogram modification and iris code into the first level high frequency sub-bands of images found by Integer Wavelet Transform (IWT) using threshold embedding technique. The watermarked-image carrying iris code is obtained after applying inverse IWT. Simply by reversing the embedding process, the original image and iris code are extracted back from watermarked-image. Authentication is done using the metric called Hamming Distance. Experimental results show that this approach outperforms the prior arts in terms of PSNR. Also, we tested with different attacks on watermarked-image for showing the sustainability of the system.
|
K. Seetharaman and R. Ragupathy, (2012) Iris Recognition based Image Authentication, International Journal of Computer Applications, Vol. 44(7), 2012, pp. 0975 – 8887 (ISSN: 0123-4560;) [ ]
|
63.
|
ABSTRACT:
In general, a typical iris recognition based Personal Identification System (PIS) includes iris imaging, iris image quality assessment, fake iris detection, and iris recognition. This paper presents a novel approach, which focusing on iris recognition. The novelty of this approach includes improving the speed and accuracy of the iris segmentation process, fetching the iris image so as to reduce the recognition error, producing a feature vector with discriminating texture features and a proper dimensionality so as to improve the recognition accuracy and computational efficiency. The Canny edge detection and circular Hough transforms are used for the segmentation process. The segmented iris is normalized using Daugman's rubber sheet model from [-32(0), 32(0)] and [148(0) 212(0)]. The phase data from 1D Log-Gabor filter is extracted and encoded efficiently to produce a proper feature vector. Experimental tests were performed using CASIAIrisV3 and UBIRIS iris databases. These tests prove that the proposed algorithm has an encouraging performance. (c) 2012 Published by Elsevier Ltd. Selection and/or peer-review under responsibility of Noorul Islam Centre for Higher Education
Procedia Engineering 01/2012; 38:1531-1546. DOI:10.1016/j.proeng.2012.06.189
K. Seetharaman and R. Ragupathy, (2012) Iris recognition for personal identification System, Proceeding Engineering, Vol.38, 2012, pp. 1531-1546 (Elsevier, ISSN:1877-7058; Scopus Indexed ). [ ]
|
64.
ABSTRACT:
This paper proposes a simple but efficient scheme for colour image retrieval, based on statistical tests of hypothesis, namely test for equality of variance, test for equality of mean. The test for equality of variance is performed to test the similarity of the query and target images. If the images pass the test, then the test for equality of mean is performed on the same images to examine whether the two images have the same attributes / characteristics. If the query and target images pass the tests then it is inferred that the two images belong to the same class i.e. both the images are same; otherwise, it is assumed that the images belong to different classes i.e. both the images are different. The obtained test statistic values are indexed in ascending order and the image corresponding to the least value is identified as same / similar images. The proposed system is invariant for translation, scaling, and rotation, since the proposed system adjusts itself and treats either the query image or the target image is sample of other. The proposed scheme provides cent percent accuracy if the query and target images are same, whereas there is a slight variation for similar, transformed. Keywords: Variance, Mean, Query Image, Target Image, Tests of Hypothesis
|
K. Seetharaman and T. Hemalatha, (2011) A Simple But Efficient Scheme for Colour Image Retrieval Using Statistical Tests of Hypothesis, Journal on Image and Video Processing, Vol. 1(3), 2011, pp. 166-171 (ISSN: 0976-9099). [ ]
|
65.
ABSTRACT:
In this paper, a novel technique is proposed based on a Family of Full Range Autoregressive (FRAR) models to extract edges in 2D monochrome images. The model parameters are estimated based on Bayesian approach and is used to smooth the input images. At each pixel location, residual value is calculated by differentiating the original image and its smoothed version. Edge magnitudes and its directions are measured based on the residual. The edge magnitudes are squared to enhance the edges whereas the other values are suppressed by using confidence limit is based on the global descriptive statistics. Threshold value is fixed automatically based on the autocorrelation value calculated on the smoothed image. This extracts the thick edges. To obtain thin and continuous edges, the nonmaxima suppression algorithm is applied with the confidence limit based on the local descriptive statistics. Then the performance of the proposed technique is compared with that of the existing standard algorithms including Canny's algorithm. Since Canny's algorithm oversmoothes across the edges, it detects the spurious and weak edges. This problem is overcome in the proposed technique because it smoothes minimally across the edges. The extracted edge map is superimposed on its original image to justify that the proposed technique is locally characterize the edges correctly. Also, the proposed technique is experimented on synthetic images such as concentric circle and square images to prove that it detects the edges in all directions and edge junctions.
|
K. Seetharaman and R. Krishnamoorthi, (2007) A Statistical Framework Based on A Family of Full Range Autoregressive Models for Edge Extraction, Pattern Recognition Letters, Vol. 28(7), 2007, pp. 759-770 (Elsevier, ISSN: 0167-8655; Impact Factor:2.810; Scopus Indexed ).
[ ]
|
66.
ABSTRACT:
In this paper, we propose a family of stochastic models for image compression, where images are assumed to be Gaussian Markov random field. This model is based on stationary full range autoregressive (FRAR) process. The parameters of the model are estimated with the Monte-Carlo integration technique based on Bayesian approach. The advantage of the proposed model is that it helps to estimate the finite number of parameters for the infinite number of orders. We use arithmetic coding to store seed values and parameters of the model as it gives furthermore compression. We also studied the use of Metropolis-Hastings algorithm to update the parameters, through which some image contents such as untexturedness are captured. Different types-both textured and untextured images-are used for experiment to illustrate the efficiency of the proposed model and the results are encouraging
|
R. Krishnamoorthi and K. Seetharaman, (2007)Image Compression Based on A Family Of Stochastic Models, Signal Processing, Vol. 87(3), 2007, pp. 408-416 (Elsevier, ISSN: 0165-1684; Impact Factor:4.086; Scopus Indexed ).
[ ]
|
67.
ABSTRACT:
Abstract content goes here..
|
R. Krishnamoorthy and K. Seetharaman, (2005) Image Compression Using 2-D Autoregressive Model, Ultra Scientist of Physical Sciences - An International Journal of Physical Sciences, Vol. 17(3)M, 2005, pp. 343-350 (ISSN: 0970-9150).
[ ]
|
|
|
|